Colorful spectrum lights with black background. 8k resolution. Best for wide banner, poster, header website, social media, editing video, background presentation, promotion and more | Image Credit: © Rijaliansyah - stock.adobe.com.
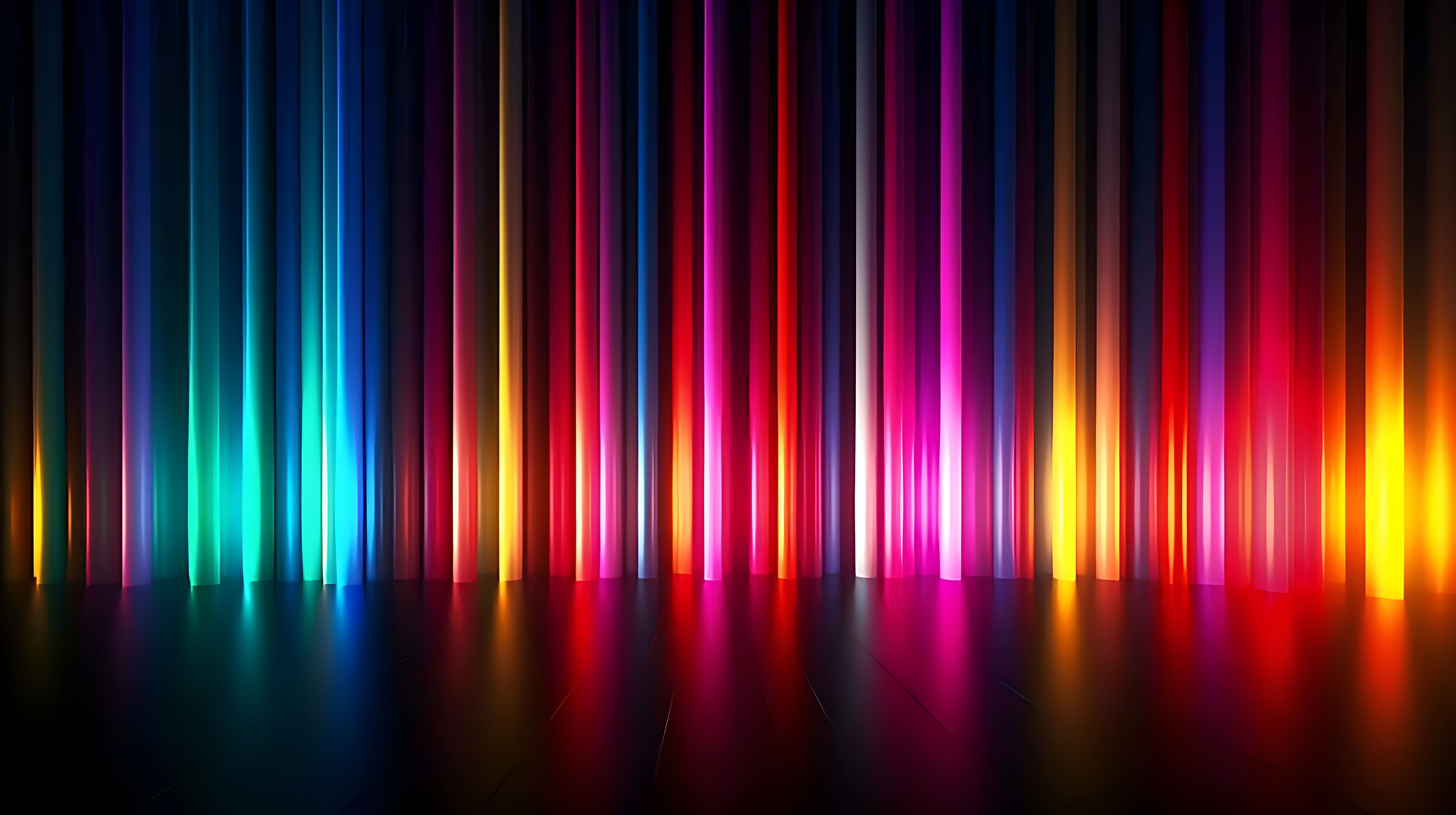
In a groundbreaking study published in Applied Spectroscopy, researchers Sicen Dong, Yuping Liu, Hanxiang Yu, Yuqing Wang, and Junchi Wu, from the College of Physics and Optoelectonic Engineering at the Harbin Engineering University in Harbin, China, introduce an innovative approach to baseline correction for Raman spectra. Their paper, titled "An Iterative Curve-Fitting Baseline Correction Method for Raman Spectra Driven by Neural Network," presents a novel method that employs a neural network model to enhance the accuracy of baseline correction.
Colorful spectrum lights with black background. 8k resolution. Best for wide banner, poster, header website, social media, editing video, background presentation, promotion and more | Image Credit: © Rijaliansyah - stock.adobe.com.
Baseline correction is a crucial step in spectral preprocessing, particularly for Raman spectra. While iterative polynomial fitting has been a common method, it is deemed less accurate compared to alternative techniques like wavelet transform and penalized least squares (PLS) methods. The drawbacks include potential distortions in results under certain conditions.
The proposed neural network model takes a distinctive approach by dynamically selecting the function basis based on the baseline trend, deviating from the conventional fixed polynomial functions. This results in a more precise fit, especially in cases where the baseline exhibits unusual shapes. The researchers also introduce a method for generating simulation data, crucial for training the neural network model, which subsequently produces reliable results for real spectral data, even in the presence of noise.
The iterative process involves taking a raw spectrum and deriving a neural network model that suggests a fitting vector, providing guidance for correcting the spectrum in each iteration. Once the neural network iterations are complete, the corrected spectrum is generated, showcasing the method's efficacy in improving baseline correction accuracy.
To further validate their approach, the researchers detail how the data and code file can be used to test their method. The entire data-generation process is executed in Rstudio, demonstrating that the method can generate various baselines with different trends. The intensity of the simulation data, ranging from 0 to 1, is crucial for model training. The researchers opted not to upload the entire dataset due to its size, but the model-training process in Python, utilizing the Pytorch package, is thoroughly explained.
In addition to revolutionizing Raman spectra analysis, the study critically evaluates the limitations of conventional iterative polynomial fitting and adaptive iteratively reweighted PLS methods, elucidating why the proposed neural network-driven approach outperforms these methods.
This truly novel research approach marks a significant advancement in the field of spectroscopy, offering a new paradigm for baseline correction in Raman spectra analysis. The implications of this study extend to various scientific disciplines relying on precise spectral data analysis.
This article was written with the help of artificial intelligence and has been edited to ensure accuracy and clarity. You can read more about our policy for using AI here.
(1) Dong, S.; Liu, Y.; Yu, H.; Wang, Y.; Wu, J. An Iterative Curve-Fitting Baseline Correction Method for Raman Spectra Driven by Neural Network. Appl. Spectrosc. 2023, First published online December 6, 2023. https://doi.org/10.1177/0003702823121294.
Get essential updates on the latest spectroscopy technologies, regulatory standards, and best practices—subscribe today to Spectroscopy.
AI-Powered Raman with CARS Offers Laser Imaging for Rapid Cervical Cancer Diagnosis
July 15th 2025Chinese researchers have developed a cutting-edge cervical cancer diagnostic model that combines spontaneous Raman spectroscopy, CARS imaging, and artificial intelligence to achieve 100% accuracy in distinguishing healthy and cancerous tissue.
Nanometer-Scale Studies Using Tip Enhanced Raman Spectroscopy
February 8th 2013Volker Deckert, the winner of the 2013 Charles Mann Award, is advancing the use of tip enhanced Raman spectroscopy (TERS) to push the lateral resolution of vibrational spectroscopy well below the Abbe limit, to achieve single-molecule sensitivity. Because the tip can be moved with sub-nanometer precision, structural information with unmatched spatial resolution can be achieved without the need of specific labels.
sCMOS vs CCD: Advancing High-Speed CARS Spectroscopy
July 14th 2025A new comparative study shows that scientific CMOS (sCMOS) cameras could rival traditional CCD detectors in certain Raman CARS spectroscopy applications, offering faster readout and dynamic range despite slightly higher noise levels.